AI vs. Generative AI: What's the Difference?

Artificial intelligence (AI)-powered tools are becoming a crucial part of the legal process. In fact, the legal tech market is projected to reach $32.54 billion by 2026, illustrating the sector's rapid growth. Additionally, AI adoption in law firms skyrocketed from 19% in 2023 to 79% in 2024, a clear indication that firms will only continue to embrace this technology.
But with terms like generative AI, machine learning, and large language models (LLMs) often used interchangeably, it’s easy to lose sight of what these technologies actually do and how they differ.
Phillip Isola, Associate Professor of electrical engineering and computer science at MIT, explains:
“When it comes to the actual machinery underlying generative AI and other types of AI, the distinctions can be a little bit blurry. Oftentimes, the same algorithms can be used for both."
While attorneys don’t necessarily need to master the technical details, understanding the basic differences between traditional AI and generative AI offers helpful context for evaluating the tools being introduced into their workflows for tasks like discovery, case strategy, or plaintiff outreach, for example.
This article unpacks those distinctions, explains how each type of AI works, and explores their growing presence in legal technology, all through the lens of how these tools actually help deliver better legal outcomes.
What is Artificial Intelligence?
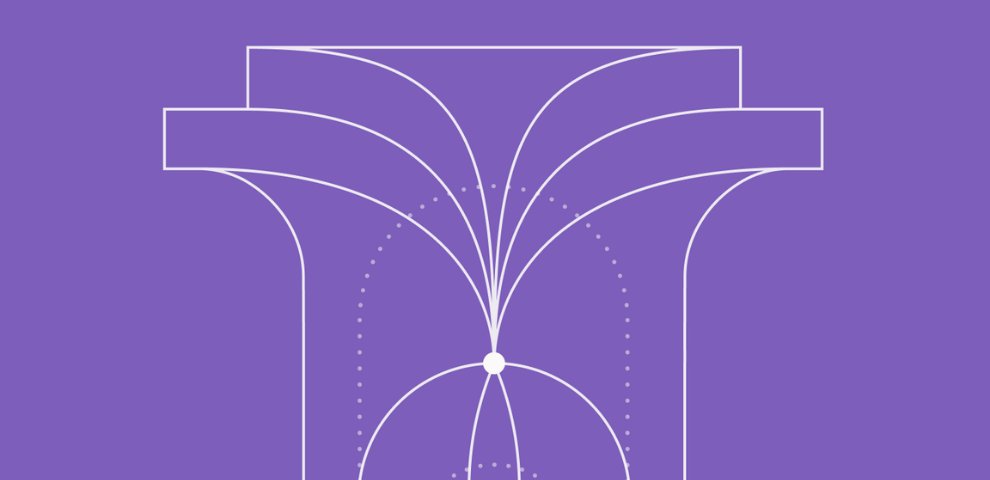
Artificial Intelligence, or AI, refers to computer systems that are built to perform tasks typically requiring human intelligence. These tasks include learning from data, recognizing patterns, making decisions, and automating workflows. In law, traditional AI is typically used to classify, sort, and prioritize large volumes of information. It operates based on programmed logic or learned behaviors, excelling at high-volume, repetitive tasks.
For example, many plaintiff firms use AI during discovery. Tools that automatically tag responsive documents, identify privileged communications, or sort by relevance are powered by traditional AI models. These systems rely on historical data and predetermined logic to categorize information quickly and consistently.
AI can also support predictive analytics. AI models can be trained on past outcomes to help assess the likelihood of success in a particular jurisdiction or with a specific judge. While not foolproof, these tools are valuable for managing risk.
What traditional AI does not do is generate new content, synthesize abstract arguments, or operate flexibly when inputs change. It follows patterns rather than interpreting nuance.
What is Machine Learning?
Machine learning (ML) is a method used within artificial intelligence that allows software to improve its performance over time by learning from data. Instead of being explicitly programmed to perform every task, a machine learning model is trained on large datasets and develops the ability to make predictions, identify patterns, or classify information based on what it has seen before.
In the legal field, many tools described as AI-powered are driven by machine learning. For example, an ML model might be trained on thousands of legal documents to identify which are likely to be relevant to a particular issue, or which phrases indicate attorney-client privilege. The model improves as it sees more examples, refining its accuracy based on patterns it learns in the background.
Machine learning is particularly useful in areas where large datasets can inform predictions or classifications. This can include:
- Predictive coding during discovery
- Case outcome prediction based on jurisdiction and judge history
- Contract review systems that flag non-standard clauses
- Legal research engines that surface the most relevant precedents
While ML is a foundational technology behind many legal tech tools, it doesn’t generate new content, offer explanations, or interpret open-ended questions. That’s the role of generative AI, which builds on some of the same methods but goes a step further.
What is Generative AI?
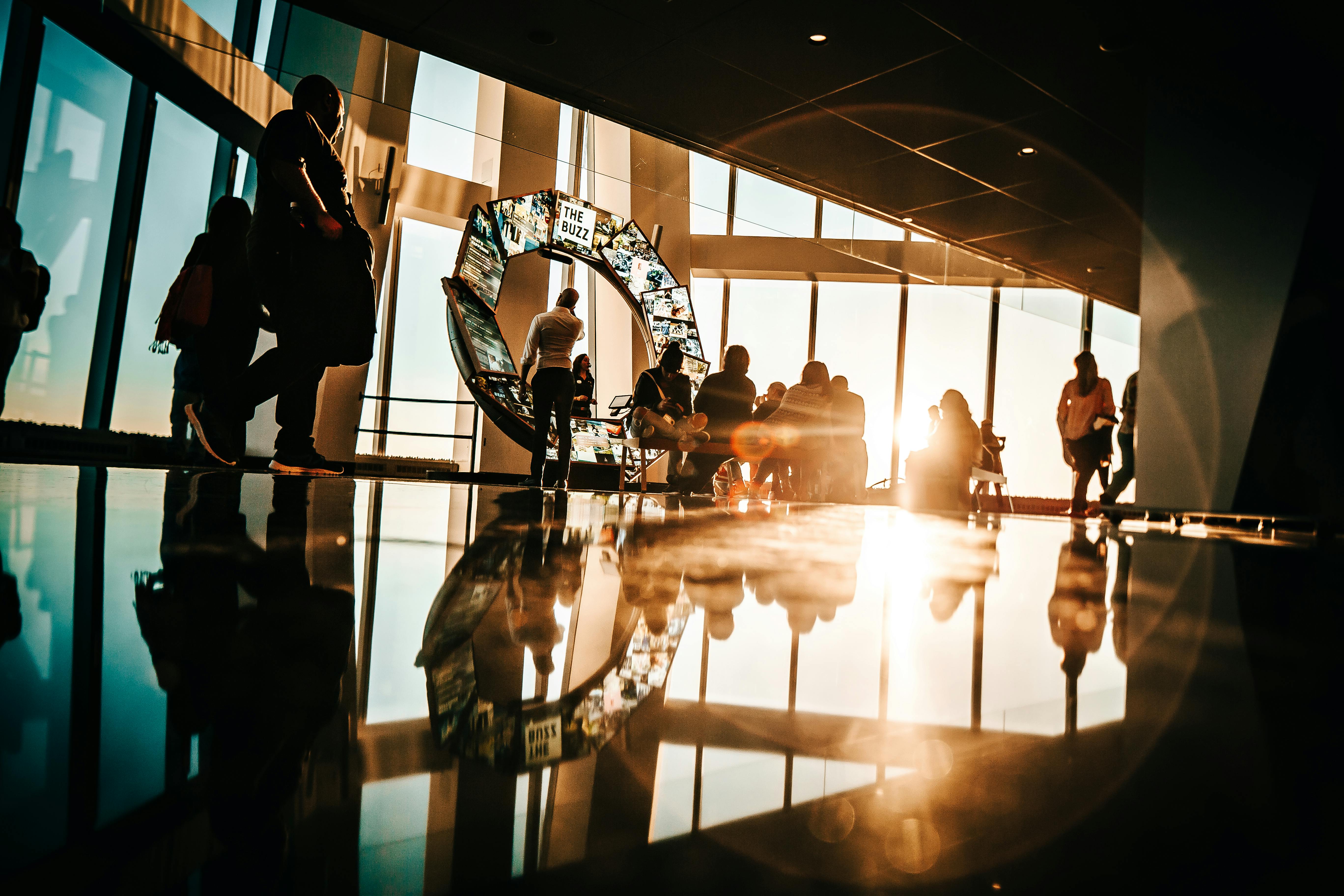
Generative AI (GenAI) refers to a subset of artificial intelligence that is usually built on machine learning models trained to generate new content. While traditional machine learning is often used to classify or predict based on existing data, generative models create entirely new outputs based on patterns they’ve learned during training.
Early experiments with generative AI relied on a method called Markov chains, which generate new content by predicting the next item in a sequence based only on the current state, without broader context. For instance, a simple Markov model trained on the phrase “the cat in the hat” might produce repetitive output like “the cat in the cat in the cat” because it selects each next word based only on the one that came before it, not on the full structure or meaning of the sentence.
While this approach could generate short, locally coherent sequences, it often broke down when trying to produce meaningful or grammatically correct text over longer stretches. This limitation helped pave the way for more advanced models, such as recurrent neural networks (RNNs) and transformers, that can track much larger amounts of context and produce more sophisticated results
Today's GenAI outputs can include text, images, audio, video, or even computer code. In contrast to tools that sort or tag data, generative AI can produce original content that mimics human input.
Generative AI includes various model types, each specialized in producing different forms of content:
- Large language models (LLMs) generate and understand human language.
- Text-to-image models generate images based on written descriptions.
- Text-to-audio models synthesize human-like speech from written input.
- Code generation models assist in writing or debugging software code based on prompts.
- Video generation tools are beginning to produce short video clips from text or image inputs.
While there are many types of GenAI, in the legal world, generative AI most often takes the form of text generation powered by LLMs. These include OpenAI (GPT), Anthropic (Claude), and others, as well as models specifically fine-tuned for legal use cases.
Understanding LLMs
LLMs can take a prompt, question, or partial input and produce a full-length response in natural language. For example, a plaintiff attorney might input a brief summary of a case and ask a generative model to draft a demand letter, write an issue summary, or generate deposition questions. These tools are context-aware and capable of adjusting tone, length, and structure depending on the task.
Unlike traditional AI, LLMs are dynamic: they can synthesize concepts and produce language that feels conversational, often closely mimicking human writing. This makes it a powerful tool for research, drafting, summarizing, and even brainstorming. However, LLMs do not inherently understand legal precedent or jurisdictional nuances unless specifically trained on relevant legal data.

At Darrow, for example, generative AI helps bridge the gap between raw data and actionable insight. Darrow’s Legal Intelligence Analysts use pre-trained LLMs to process vast amounts of long documents quickly and at scale. Our technology allows the team to process massive amounts of information, identify patterns, cluster related data, and draw actionable conclusions while ensuring that human legal experts remain central to interpreting results and validating conclusions.
LLMs evaluate data and rank potential legal risks, prioritizing those with the most substantial evidence for building strong cases. This allows us to maximize efficiency and ensure we focus our resources to uncover potential legal violations with speed and precision.
We send structured questions tailored to the specific information we need, and the model answers each piece of data we analyze, significantly accelerating the detection of legal risk and evidence collection.
Learn more about Darrow’s use of LLMs to detect hidden legal violations: How LLMs are Reshaping the Legal Industry
However, if not used responsibly, LLMs can pose risks. These machines have the ability to produce inaccurate or outdated information if not trained properly, especially when asked legal questions that require jurisdiction-specific knowledge. The outputs are only as good as the underlying training data and prompts, and they require human oversight to validate quality and accuracy.
Choosing Legal Tech Tools with Outcomes in Mind
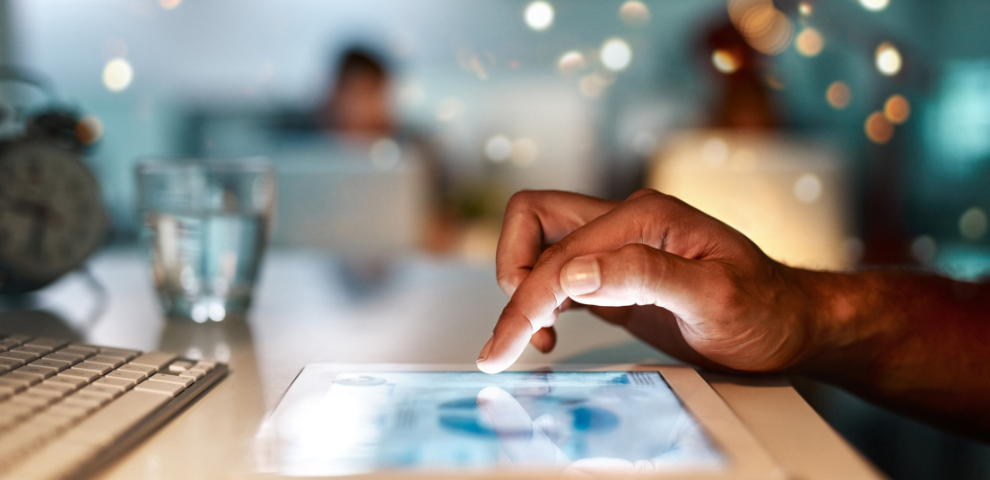
Understanding the technical differences between traditional AI, machine learning, and generative AI is important, but these definitions alone don’t tell the full story. The real question for attorneys isn’t just how these tools work, but how they can help achieve the outcomes that matter most for clients.
This is where the thinking of Professor Richard Susskind, chair of the Law Chief Justices’ AI Advisory Group and a leading voice in legal innovation, offers valuable perspective. In a November 2024 lecture at the University of Liverpool, he argued that while understanding how AI legal tools function is important, the real focus shouldn’t be on how lawyers work today, but on the outcomes clients actually need.
Susskind calls this approach outcome thinking. It challenges the common assumption, what he terms the AI fallacy, that technology must replicate human reasoning to be effective. Instead, the real question is not How do we automate lawyers? but How do we use technology to deliver better legal outcomes, faster, cheaper, and at scale?
As Susskind puts it:
“The question is not what’s the future of lawyers, but how in the future will we solve the problems to which today lawyers are the best answer.”
In other words, the value isn’t in mimicking lawyers, but in amplifying their capacity to achieve the results that matter most for clients.
When legal tech is guided by outcome thinking, the focus shifts from automating tasks to enhancing judgment, improving access to justice, and accelerating case development. This mindset helps ensure that both traditional and generative AI tools are deployed where they can make the greatest real-world impact, not just where they seem most impressive.
Justice Through Intelligence: How Darrow Uses AI to Detect Legal Violations
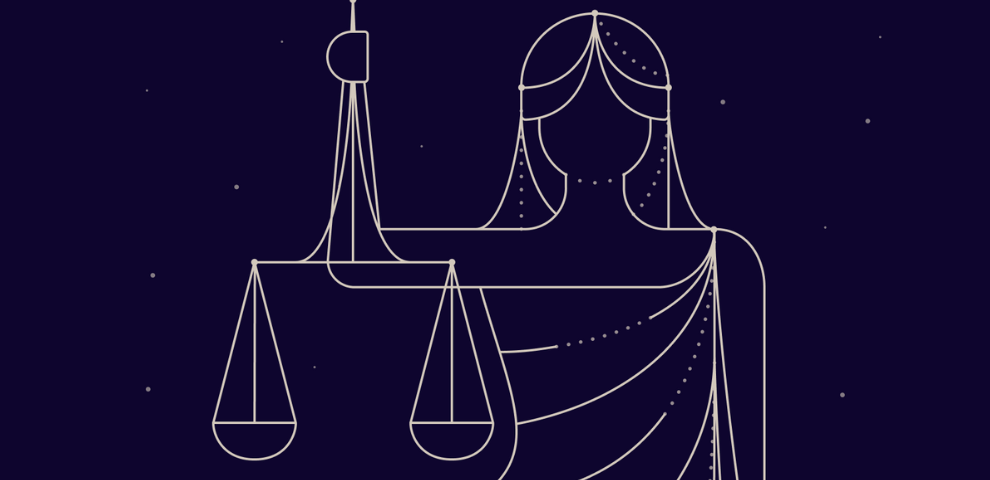
Professor Susskind’s concept of outcome thinking aligns with Darrow’s mission of justice through intelligence. At Darrow, the aim is not to automate legal work for its own sake, but to uncover hidden legal violations, empower attorneys, and help bring justice to those who need it most.
This mission shapes how we develop and apply technology. Our legal intelligence infrastructure combines traditional AI, generative AI, data analytics, intelligence work, and human expertise to turn raw information into actionable insight. We develop technology to amplify legal judgment, not replace it. Attorneys at partner firms use these insights to develop well-supported cases, backed by robust evidence, legal context, and documentation ready for litigation.
This Might Interest You: